In the realm of B2B marketing, the journey from capturing a lead to converting it into a sale is akin to nurturing a seed into a blooming plant. It requires patience, dedication, and the right strategies to ensure growth. This is where lead nurturing comes into play. This process, when executed effectively, can produce a 20% increase in sales opportunities compared to non-nurtured leads. Yet, as our digital landscape expands and customer expectations rise, personalizing each lead’s journey while scaling operations becomes a challenge.
The Foundations of Lead Nurturing
Lead nurturing is essentially the process of developing relationships with buyers at every stage of the sales funnel. It focuses on marketing and communication efforts on listening to the needs of prospects and providing the information and answers they need. However, traditional nurturing efforts faces several challenges, including how to provide personalized attention at scale and how to accurately target leads ready for sales engagement.
Why Lead Nurturing is Essential for B2B Success
The statistics paint a clear picture: nurtured leads not only enhance the sales pipeline but also have a significant impact on the business’s bottom line. Around 96% of prospects who visits your website are not ready to buy yet before getting nurtured . But the benefits extend beyond numbers, fostering trust and establishing lasting relationships with potential customers.
Embracing AI for Superior Lead Nurturing
This is where Artificial Intelligence (AI) steps in, transforming nurturing endeavors from a tiring task into an efficient and highly personalized process. In general, AI technologies allows businesses to analyze vast amounts of data and generate great number of content and personalized messages with lesser effort. This automatization allows businesses to tailor and allocate their efforts more accurately.
Strategies for AI-Assisted Lead Nurturing
Segmenting Leads with Precision
In B2B, lead segmentation is necessary to better categorize potential customers based on various attributes so that marketing efforts can be more accurately targeted. With AI, this process can be much more effective by introducing a high level of precision at a large scale. Here’s how it is possible:
Advanced Data Analysis
AI tools use advanced algorithms that can sift through massive datasets much more efficiently than humans. They can detect subtle patterns and correlations among data points that might not be immediately obvious. This capability allows the process of data analysis to progress much faster as it narrows down information from massive data to ones that you can reliably use.
Real-time Segmentation
One of the standout features of AI in lead segmentation is its ability to process data in real-time. This means that with the right setting and AI- learning, as soon as new data comes in—be it from a lead’s interaction on a website or a social media engagement—AI can instantly categorize the lead into the appropriate segment. This dynamic segmentation ensures that marketing efforts are always targeted based on the most current understanding of a lead’s preferences and intent.
Behavioral and Contextual Segmentation
Beyond traditional segmentation criteria (like demographics), AI can segment leads based on how they interact with your business and the context of those interactions. For example, AI can analyze web browsing behavior, purchase history, and even the time and device used for interactions to create detailed profiles that inform more personalized marketing strategies.
Personalizing Communications at Scale
Not only for shifting through data, AI can also assist in the creation of personalized content. AI-driven tools allows the creation of emails, messages, and content without needing to manually craft each message. This makes personalization at scale not only possible but practical, as it is no longer required to start from scratch when making personalized message; you just need to revise an existing initial draft that the AI have generated. Not to mention, with improvement in AI-technology in the future, a natural personalized message without the need of manual edits might be possible.
Predictive Lead Scoring
Before, the most automatization that marketers could do for lead scoring is by putting data of prospects’ behaviors in a sheet. With the use of AI, their algorithms can predict which leads are most likely to convert based on current data and how the model was taught. This helps sales teams prioritize their efforts and engage with leads that have the highest potential for sales success. What would the process look like? Lets look into it together!
Collecting Data
As effective as AI is, they really need data to function. This is why gathering a vast amount of information on potential customers; whether it’s from ones already accessible in your database or ones that are newly gathered is important. Here are some example data that you could use for AI-learning:
- Preferences
- Online behavior
- Email interactions
- Social media interactions
- Website activity
This creates a comprehensive profile for each prospective client that the AI model can use in their process of lead scoring later on.
Identifying Key Indicators
Of course, just having the data is not enough. The AI model would also need to know what are the key indicators that show a prospect is leaning towards purchase. This is why it is important to list out which pieces of collected data can provide the best insight into a lead’s likelihood to make a purchase. To find this out, ask the following questions:
- Which behaviors signal strong buying intent?
- How does engagement with content correlates with purchasing?
- Why is the demographic information important?
- What are the crucial variables that the model need?
Training the AI Model
After identifying the key indicators, it is employed to machine learning algorithms that:
- Are fed historical data to ‘learn’ what successful conversions look like
- Can distinguish between high and low potential leads based on this data
This is a crucial step as it is where the AI model really starts to take shape. If it’s not trained properly to be able to distinguish which leads can lead to successful conversions, it may ended up being counter-productive. We don’t want the AI to give us the wrong leads to focus on instead, do we?
Implementing Lead Scoring
With the training complete, the AI model can begin to:
- Assigning scores to new leads
- Ranking them according to their likelihood of conversion
Sales teams can then prioritize their efforts toward the most promising leads.
Ongoing Evaluation and Adjustment
As previously mentioned, the wrong training of AI can lead to counter-productive efforts. This is why maintaining the model’s effectiveness is needed in the ever changing market climate. This maintenance involves:
- Regularly comparing predictions with actual outcomes to refine its accuracy
- Updating the model with fresh data to keep up with market trends
By maintaining AI-model to stay accurate and up-to-date with the current market changes, the leads that it score will continually provide effective results.
So, Lets just Leave it All to AI?
No! We can’t just leave everything or even most of the lead nurturing process to AI (at least with its current capabilities). One of the biggest pitfalls after the advent of AI is businesses’ over-reliance to it. While AI is really good at analyzing massive data sets and preparing initial drafts for personalized messages, errors are still very likely. Here are some errors that may happen with AI use for lead nurturing:
Data quality issues
- Inaccurate Data: AI systems rely on the input data to learn and make decisions. If the data is inaccurate, it can lead to incorrect predictions.
- Incomplete Data: Missing information can cause the AI to overlook potential leads or misjudge their quality.
Bias in the AI
- Algorithmic Bias: The AI might develop biases based on historical data, reflecting past decision-making, which may not be suitable for the present context.
- Data Bias: If the training data is not representative of the entire target audience, the AI’s lead scoring may favor certain groups over others.
Overfitting and Underfitting
- Overfitting: An AI model might perform exceptionally well on the training data but fail to generalize to new data.
- Underfitting: The AI model might be too simple and unable to capture the complexity of lead behaviors, resulting in poor performance.
To mitigate these issues, it is important to regularly audit and update AI systems, ensure diversity in training data, and involve stakeholders in the development process. This allows the AI to stay free of errors and can be continuosly used.
In an era where personalization and efficiency are what people are going for, AI-assisted lead nurturing offers a beacon of hope for both B2B marketers and sales professionals. By embracing AI, businesses can not only meet but exceed their sales and marketing objectives, forging stronger connections with their audience and driving unparalleled growth. However, it is important to keep in mind that an over-reliance on something can be a bad thing. Use of AI comes with the need to perpetually maintain and updating it. Forgetting that we are in control over the AI and not the other way around can lead to counter-productive results!
Like what you’re reading so far? We post content like this every week! If you’re interested in more insights on B2B Digital Marketing, Lead Generation and Lead Nurturing, come visit us! Click here to get started!
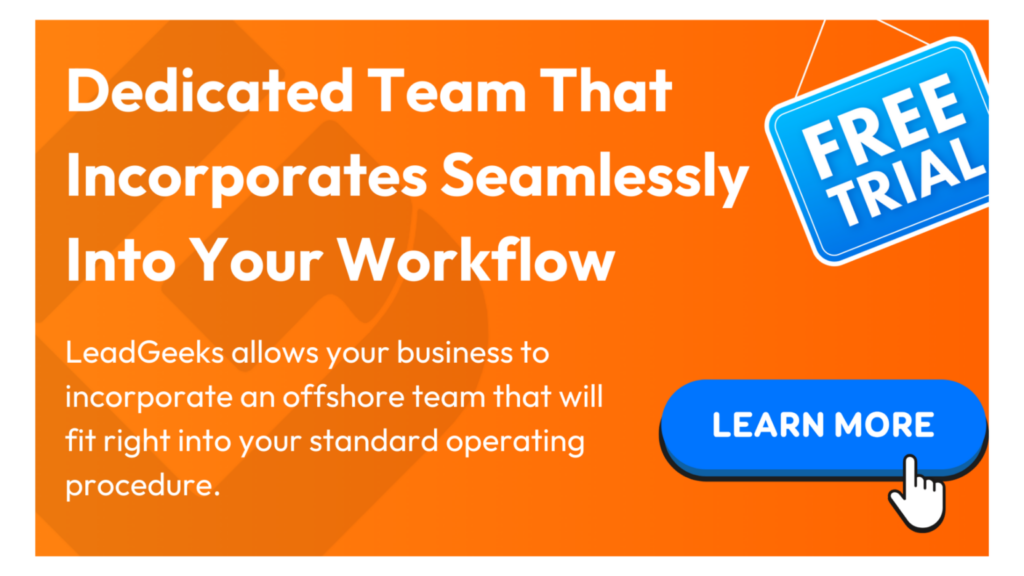
Leave a Reply