Imagine navigating a ship in the dark without a compass. This scenario mirrors businesses operating without the insights derived from robust sales data. In today’s data-driven landscape, understanding and utilizing sales data can significantly transform decision-making processes and competitive strategies. This blog post will delve into why sales data is indispensable, how to efficiently gather and organize it, and most importantly, how to analyze and leverage it for optimal business growth.
What Exactly is Sales Data?
At its core, sales data encompasses the myriad pieces of information that are harvested from various sales-related activities. This data doesn’t just tally up your sales; it’s a rich tapestry that includes customer transactions, sales volumes over specific periods, and the frequency of purchases, to name a few. However, it’s much more than mere numbers and charts. Sales data is a reflection of your business’s heartbeat, providing a pulse on customer behaviors, preferences, and how they interact with your brand over time.
Why Is Sales Data Important?
In the vibrant tapestry of market trends, sales data acts as the linchpin, a critical connector that aligns business operations with customer expectations and market dynamics. It serves as a beacon, guiding businesses through the ever-changing landscapes of consumer behavior and market trends. By analyzing sales data, businesses can decipher patterns, predict future purchasing behaviors, and tailor their strategies to meet the market’s pulse. This intelligence allows for strategic decisions that are not based on hunches or assumptions but grounded in factual evidence and trends discerned from actual sales data.
From Intuition to Informed Decision-Making
Rely reliance on intuition is akin to navigating a ship through fog with a broken compass. While intuition may play a role in the initial stages of a business, reliance on gut feeling alone is untenable for sustained growth and success. By using sales data, you will have a factual basis for decisions that affect every aspect of your business from product development to marketing strategies.
Sales data minimizes guesswork and amplifies clarity. It informs you of what products are flying off the shelves and which ones are not resonating with your audience. It tells you when to ramp up marketing efforts and when to pivot. For any business that aspires to not just survive but thrive in today’s cutthroat market, understanding and leveraging sales data is not just important—it’s imperative.
Sources and Types of Sales Data
Sources of Sales Data
CRM Systems
CRM (Customer Relationship Management) systems are akin to the alchemists of the digital era, transforming raw customer interactions into gold mines of data. These systems meticulously record every interaction with customers, from emails and sales calls to social media engagements and beyond. Imagine holding a detailed script of every act played out on your business stage — that’s the power of CRM data.
Customer Feedback
Direct feedback from customers, whether harvested through surveys, reviews, or social media, serves as an unfiltered lens into the heart and soul of your audience’s desires and grievances. Like a skilled therapist, your role is to listen, interpret, and act on this feedback, turning insights into action.
Point of Sale (POS) Systems
POS systems do more than process payments; they are the narrators of every purchase story. This source is particularly rich in transactional data, detailing what items are selling like hot cakes, the average spend per customer, and peak shopping hours. Each transaction recorded is a verse in the epic of your market’s behavior.
Direct Customer Interactions
Direct interactions, whether in-person, via phone, emails or through online chats, offer a raw and often underutilized source of sales data. These interactions are treasure troves of qualitative data, capturing the nuances of customer concerns, questions, and preferences in a way no algorithm can.
Deciphering the Types of Sales Data
Transactional Data
Transactional data is the backbone of sales analysis, cataloging the who, what, when, and how much of every purchase. This is your quantifiable scoreboard, offering clear metrics on sales volumes, product popularity, and revenue trends.
Customer Interaction Data
This data type zooms in on how customers interact with your brand across various touchpoints. It’s akin to reading the diary of your customer’s journey, providing insights into their purchasing path, from initial interest sparked by an ad to the final decision made at checkout.
Qualitative Feedback
While numbers and charts offer clarity, qualitative feedback delves into the why. This is the data that sheds light on customer sentiment, satisfaction, and loyalty. It’s akin to sitting down for a coffee with your customers and understanding their perspectives, aspirations, and pain points.
What Sales Data Gathering Look Like
The quest for actionable sales data commences with its meticulous collection. Businesses, in their role as data custodians, must implement formidable systems for capturing and recording this invaluable information. This is no mere happenstance but a choreographed dance of strategic planning and execution.
Crafting Data Collection Guidelines
When you’re setting the stage for effective data collection, it’s critical to tailor your guidelines to the unique narrative of your business goals. Rather than collecting data aimlessly, you must identify the data that casts the leading characters in your performance analysis.
For instance, if your primary objective is to analyze sales trends, ensure your guidelines stipulate the tracking of variables such as:
- Timeframe of sales (daily, weekly, monthly)
- Amount of revenue generated per product
- Geographic location of sales
- Sales channels used (e-commerce, in-store, third-party platforms)
If customer demographics are your spotlight, you’d focus on different data points:
- Age
- Gender
- Purchase history
- Location details
- Behavioral data (browsing history, device usage, etc.)
Assessing product performance? Then these might be your stars:
- Units sold per time period
- Customer reviews and ratings
- Return rates
- Inventory levels
Your manuscript of guidelines must articulate the how — for example, using CRM software to automatically capture customer interactions — and the why — to understand purchasing patterns and tailor marketing efforts accordingly.
Ensuring Consistency in Data Collection Methods
Uniformity in data collection is the chorus that harmonizes the entire symphony. Consistent methods eliminate the dissonance of incongruous data, resulting in insights that truly resonate with the story you’re telling.
Here’s an example for clarity:
Assume two sales reps are entering details of customer interactions. One uses an automated tool that timestamps and categorizes each interaction based on keywords, while the other takes notes manually, using subjective descriptors and inconsistent time records.
The disparity in their methods will render the collected data akin to comparing an abstract with Renaissance art — both valuable but hardly cohesive. To rectify this, implement a standard CRM system across the company. Train each sales rep to record interactions using predefined dropdowns and checkboxes. This will ensure that the data from both reps will not only align but will amplify each other when analyzed together.
Implementing automation, like integrating your e-commerce platform with your CRM, ensures that sales data is transferred consistently, minimizing human error and maximizing efficiency.
By engraining these detailed guidelines and consistent collection methods into your organization’s fabric, you lay down the tracks for the train of actionable insight to run smoothly. Consistency and clarity become your powerful allies, enabling you to forge strategies with the confidence of data-driven swords, sharpened and ready for the dynamic battleground of digital marketing.
Organizing Collected Sales Data
With a treasure trove of data at hand, the challenge shifts to transforming this raw material into organized, analyzed, and actionable insights. Lets go into what you need to prepare to make this possible!
The Database: Your Digital Library
Imagine entering a grand library where every book, from timeless classics to contemporary bestsellers, is methodically organized, not merely occupying shelf space. In the digital realm, this library takes the form of a database.
For Example:
Using a CRM like Salesforce or a database like MySQL, consider how your sales data would be segmented. You’d have tables resembling bookshelves, where one holds all the information on customers (names, contact details, preferences), another for transaction history (purchase dates, amounts, product categories), and yet another for inventory levels. Choosing a database platform is akin to picking the library building that can scale with your collection – MySQL works well for a wide range of applications, while MongoDB suits those dealing with more fluid, unstructured data.
Categorizing and Tagging Processed Data
Borrowing from the organizational genius of libraries, categorizing and tagging your data makes navigation a breeze. Data categories might include customer demographics, transaction types, or product categories, while tags can provide more granular insights, like seasonal promotions or customer feedback themes. This layer of organization turns your database into a well-oiled machine, ready to deliver specific insights at a moment’s notice.
For Example:
Consider you’re running an e-commerce platform. Here, categorization might look like:
- Customer Demographics: Age, Gender, Location, etc.
- Transaction Types: Online Purchase, In-store Purchase, Returns, Exchanges
- Product Categories: Electronics, Apparel, Home Goods, etc.
Adding tags provides further granularity. For a Spring Sale, tagging each relevant transaction allows for swift identification of trends or customer engagement with the promotion. Thus, when queried, this system effortlessly unveils insights, such as the most popular product purchased by males aged 18-24 during the sale.
Regular Maintenance and Updates:
A library without a librarian spirals into chaos. Similarly, regular audits of your data organization practices ensure the system remains intuitive and reflective of your business’s evolving needs. This might involve periodically reviewing data categories and tags for relevance, cleaning up duplicates, and updating your database software.
For Example:
Let’s say your line of wearable tech introduces a new product category – “Smart Eyewear.” Regular maintenance would involve:
- Adding New Categories and Tags: For transactions, customer feedback, and inventory related to Smart Eyewear.
- Reviewing Existing Data: Ensuring “Smart Watches” and “Fitness Trackers” aren’t erroneously tagged or categorized under the new product line.
- Software Updates: Implementing the latest database software updates to enhance security and data processing capabilities.
Additionally, duplicate record cleanup, like consolidating customer profiles accidentally created twice, ensures your database remains pristine and functional.
Cleaning and Preparing Your Sales Data
Now that your data is all gathered and organized, you’ll notice that data integrity is paramount. Cleaning data involves removing duplicates, correcting errors, and filling in missing values. Lets go into some steps on how you can ensure your data stays clean and ready to use.
Step 1: Identifying and Removing Duplicates
Duplicates in your dataset are akin to echoes in a conversation; they add volume but no value, skewing analysis and leading to erroneous conclusions. The first step in your data cleansing odyssey is to identify and excise these redundancies.
In Google Sheets or Excel, this might involve using the “Remove Duplicates” function accessible through the Data tab. Sophisticated platforms like Python’s pandas library offer even more nuanced control with functions like drop_duplicates(), allowing for condition-based removal of duplicates.
Step 2: Correcting Errors
Human error, system glitches, or misaligned integrations can introduce inaccuracies into your data—everything from misspelled customer names to incorrect transaction dates. This stage is akin to editing a draft, where meticulous attention to detail can transform a rough draft into polished prose.
You can fix this by utilizing Sheet or Excel’s “Find and Replace” feature can streamline corrections for common errors. Data cleansing software also often includes validation rules or correction algorithms that standardize and correct data entries automatically.
Step 3: Filling in Missing Values
A dataset with missing values is like a map with blank spaces; it can lead you astray if you’re not careful. Determining the best approach to fill these gaps requires understanding the nature of your data and the implications of various imputation methods.
For those using Google Sheets or Excel, simple solutions might involve leveraging Excel’s functions to replace missing values with averages or medians, where appropriate for quantitative data. For more nuanced approaches, data cleansing tools and statistical software offer techniques like regression imputation or machine learning models to predict and fill missing data based on patterns within your dataset.
Analyzing Sales Data
This is where the magic happens. Analysis transforms raw data into actionable insights. Techniques can range from simple descriptive statistics that provide an overview of sales trends and patterns to complex predictive analytics that forecast future sales and market behaviors. Lets get into each of them and get started!
Descriptive Statistics
Start with the fundamentals. Descriptive statistics are your first glance at the lay of the land. They offer a snapshot of your sales data, highlighting basic patterns and trends.
- Mean and Median: These measures give you an immediate sense of your average sales volume. While the mean provides the overall average, the median can offer insights into the distribution of your sales data, especially useful for identifying skewness due to exceptionally high or low sales periods.
- Standard Deviation: Understanding the variance in your sales data helps pinpoint stability or volatility in sales patterns, essential for risk assessment.
- Distribution and Range: These metrics illuminate the breadth of your data, helping you understand the spread between the highest and lowest sales figures.
Tools like Excel are perfectly suited for these initial analyses, allowing swift calculation of these essential statistical measures.
Predictive Analytics
Venturing beyond the present and past, predictive analytics uses your historical sales data to forecast future trends, demands, and customer behaviors. Techniques such as regression analysis, machine learning models, and time series analysis are the wands and spells of this domain.
- Regression Analysis: Here, you examine the relationship between sales figures and potentially influential factors (like marketing spend, seasonality, or product pricing) to predict future sales.
- Machine Learning Models: Algorithms that learn from your data can unearth complex patterns and make predictions about future sales. Tools and platforms like Python (with libraries like Scikit-learn) or R are the workhorses here, requiring a deeper dive into programming but offering unparalleled customization and depth of analysis.
- Time Series Analysis: This is crucial for forecasting, as it considers the chronological order of data points. It helps in predicting seasonal sales trends or identifying cyclical patterns, which are invaluable for inventory planning and marketing campaigns.
Visualization
Insights buried in spreadsheets and numbers are like treasure maps without markings. Visualization tools such as Tableau or Microsoft Power BI convert your analytical findings into intuitive, interactive charts and dashboards. Imagine observing a time series forecast of your sales data, with peaks and troughs clearly showing the ebb and flow of your business through the seasons. Or, visualize a heat map revealing which products are hot sellers in which regions. These tools not only illustrate your data but also highlight relationships between different data points, turning complex analyses into understandable, actionable insights.
Mastering sales data is not merely about collecting and analyzing numbers; it’s about transforming these numbers into a strategic blueprint that drives business growth. With the steps outlined above, businesses can begin to refine their approach to sales data, turning what once was daunting into manageable, tactical, and impactful business intelligence.
Now that you understand the transformative potential of sales data, take the next step. Whether you’re refining your current strategies or just beginning to focus on data-driven decisions, every piece of data you collect and analyze is a stepping stone towards unprecedented success.
If this post has been resourceful for you so far, why not read more? We provide more insights like this in our blog! Learn more and stay up-to-date to current B2B marketing strategies by following us here.
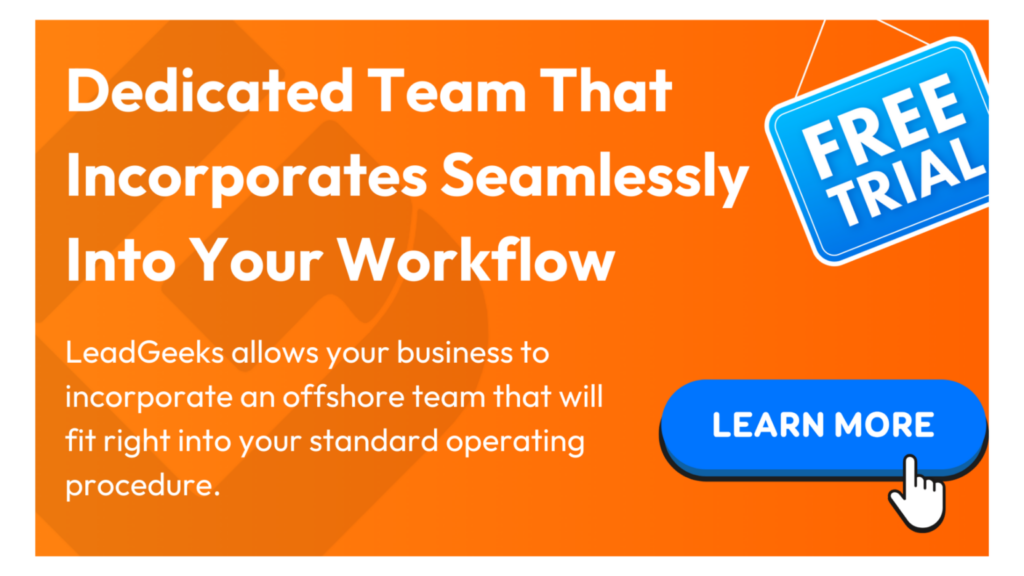
Leave a Reply