In an age when data is more than mere numbers—transforming into the lifeblood of our strategic decision-making—sales data stands out as a critical determinant of success across sectors. With businesses engulfed in an ever-evolving digital ecosystem, the call for innovation, efficiency, and foresight is loud and clear. Enter Artificial Intelligence (AI), not as a mere participant in the arena of sales data processing but as a game-changer poised to redefine paradigms.
Understanding Sales Data and AI’s Role
At its core, sales data encompasses various metrics and analyses pertaining to sales performances, customer interactions, and market trends. Yet, the sheer volume and complexity of this data often render traditional processing methodologies inadequate. This is where AI, with its capacity for machine learning, predictive analytics, and pattern recognition, comes into the limelight.
AI transforms raw data into actionable insights, enabling businesses to forecast with unprecedented accuracy, optimize sales strategies in real-time, and unlock new levels of customer engagement. But integrating AI into sales data processing is not just a matter of technology. It’s about strategically aligning AI capabilities with business objectives to revolutionize outcomes.
How AI Assist Sales Data Processing
Lets dive into how exactly AI can support the process of going through sales data. In this post, we will be touching on how AI can assist in sales forecasting and engagement.
Sales Forecasting with AI
Sales forecasting, a critical determinant of a business’s strategic planning, could greatly benefit from the precision that AI brings to the table. Lets take a closer look at how it works:
Data-Driven Insights
AI algorithms thrive on data. By ingesting vast swaths of historical sales data, including transaction records, customer interactions, and more, AI models identify patterns that human analysts might overlook. But it doesn’t stop at simple pattern recognition. Through sophisticated machine learning models, AI can weigh the impact of various external factors like market trends, economic indicators, and even sociopolitical events, offering a layered understanding of potential sales trajectories.
Predictive Modeling
Utilizing advanced statistical techniques and machine learning, AI develops predictive models that forecast future sales with a degree of precision once deemed unattainable. These models continually learn and adapt, refining their predictions as new data becomes available. For businesses, this means the capability to preemptively adjust strategies, whether it’s ramping up production in anticipation of increased demand or refining marketing tactics to address forecasted shifts in consumer behavior.
Scenario Planning
AI’s capability to simulate different scenarios based on varying inputs allows businesses to prepare for a range of outcomes. By modeling how changes in pricing, product features, or marketing campaigns could affect sales, companies can make informed decisions, minimizing risks and maximizing opportunities.
Effective Engagement with AI
While communication strictly using AI is looked down upon do to how it lacks human-touch, it is clear that AI is very good at making personalized communication more effective by integrating it with personalized engagement. Lets see how that looks!
Lead Scoring and Prioritization
AI algorithms analyze past interactions, social media activity, and other digital footprints to score leads based on their likelihood to convert. Sales representatives can then focus their efforts on those most likely to yield results, optimizing their time and enhancing productivity.
Personalized Communication
Making direct communication using AI is definitely out of the question (at least for now). However, it is still good in providing data for a more effective personalized touch. By analyzing a customer’s purchase history, preferences, and even the tone of their emails, AI tools can guide sales reps in crafting messages that resonate. This isn’t about bombarding clients with generic sales pitches that comes with just using AI, but engaging them in a meaningful dialogue that acknowledges their unique needs and preferences.
Automating Routine Tasks
The administrative aspects of sales, while necessary, can be time-consuming. AI comes to the rescue by automating tasks such as data entry, scheduling meetings, and even following up on leads. This automation not only streamlines operations but also allows sales teams to devote more time to strategic activities.
Challenges in Implementing AI for Sales Data
Despite its transformative potential, the path to integrating AI in sales data processing is fraught with challenges. Data privacy concerns, the complexity of AI systems, and the need for skilled personnel are but a few hurdles businesses might encounter. Lets have a look into each of these challenges and how to overcome them.
Data Privacy Concerns
Guarding the sanctity of data privacy is akin to safeguarding the most precious of treasures in the digital age. To address this critical concern, a meticulous approach is warranted:
- Regulatory Compliance. Ensuring strict adherence to data protection regulations, such as GDPR and CCPA, is not optional but fundamental. This acts as the moral compass guiding the organization’s data practices.
- Privacy-by-Design. Incorporating privacy into the fabric of AI systems from the very inception is a mark of prudence. It means considering privacy implications at each stage of development and deployment.
- Making Data Anonymous. By stripping away personally identifiable information (PII), companies can reduce the risk of compromising individual privacy. This allows the AI to feast on rich data without the indigestion of ethical dilemmas.
AI Systems Complexities
Between setting it up, training it and using it effectively, AI can be quite complex in its development. It requires some level of technological knowledge to get it to work. Here are some things you can do to overcome this hurdle:
- Technology Audit. A rigorous assessment of existing infrastructure sets the stage, determining the capacity to support complex AI operations. Simplify certain information that are too technical. Your team only needs to know how it affect their work and how to use it effectively.
- Modular Architecture. Adopting a modular approach in developing AI systems facilitates manageable increments. Involve your team with essential parts of how it works during its development to prepare them for when it is utilized.
- Integration Strategies. Ensure that AI systems can seamlessly communicate with legacy systems and data repositories. Properly communicate with the team how exactly the AI will affect existing systems and how it will interact with collected data.
Leveraging AI in sales data processing is no longer an option but a necessity in the digital age. As we stand on the cusp of a new era of sales strategy, the power of AI to transform data into strategic insights cannot be overstated. While there are challenges and often crucial mistakes in using AI for sales data processing, making it work is worth it.
Remember, the journey to AI integration in sales is a marathon, not a sprint. It requires strategic planning, commitment, and a willingness to evolve. But for those who dare to embrace it, the rewards are boundless—a future of unparalleled efficiency, insight, and competitive advantage awaits. As we conclude this exploration into the transformative potential of AI in sales data processing, we encourage you to embark on this journey with an open mind and a strategic vision.
If this post has been resourceful for you so far, why not read more? We provide more insights like this in our blog! Learn more and stay up-to-date to current B2B marketing strategies by following us here.
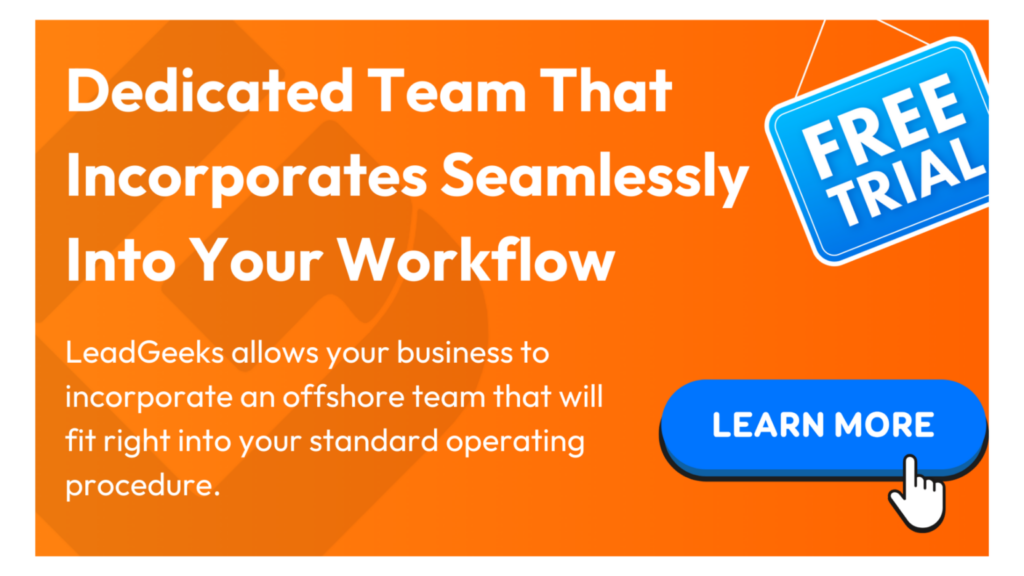
Leave a Reply